Statistical Parametric Mapping
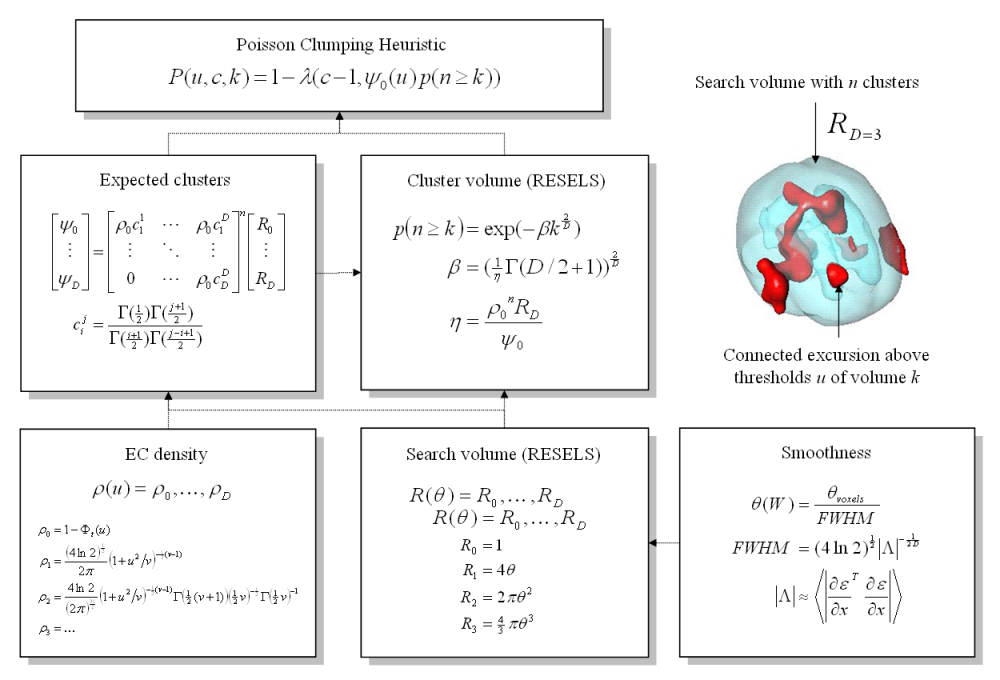
Schematic illustrating the use of Random Field Theory in making inferences about SPMs. If one knew precisely where to look, then inference can be based on the value of the statistic at the specified location in the SPM. However, generally, one does not have a precise anatomical prior, and an adjustment for multiple dependent comparisons has to be made to the p-values. These corrections use distributional approximations from RFT. This schematic deals with a general case of n SPM{t} whose voxels all survive a common threshold <img class='tex' src="/wiki/images/math/1e0fbb458ac1325b073be7a4fad98fc8.png" alt="u" /> (i.e. a conjunction of <img class='tex' src="/wiki/images/math/30c2a24cd38b0414fae013c0d44b0ca9.png" alt="n" /> component SPMs). The central probability, upon which all peak, cluster or set-level inferences are made, is the probability <img class='tex' src="/wiki/images/math/802c4d6b0b186aa9ab1853dfa96590b2.png" alt="P(u,c,k)" /> of getting <img class='tex' src="/wiki/images/math/2d043a3ca0862ca27fb2ff3a1eb2f1cf.png" alt="c" /> or more clusters with <img class='tex' src="/wiki/images/math/524eecc1d5922a435e8e848094405caa.png" alt="k" /> or more RESELS (resolution elements) above this threshold. By assuming that clusters behave like a multidimensional Poisson point-process (i.e., the Poisson clumping heuristic), <img class='tex' src="/wiki/images/math/802c4d6b0b186aa9ab1853dfa96590b2.png" alt="P(u,c,k)" /> is determined simply: the distribution of <img class='tex' src="/wiki/images/math/2d043a3ca0862ca27fb2ff3a1eb2f1cf.png" alt="c" /> is Poisson with an expectation that corresponds to the product of the expected number of clusters, of any size, and the probability that any cluster will be bigger than <img class='tex' src="/wiki/images/math/524eecc1d5922a435e8e848094405caa.png" alt="k" /> RESELS. The latter probability depends on the expected number of RESELS per cluster <img class='tex' src="/wiki/images/math/3dc2e0d31e939dc8e285bb3aa56234db.png" alt="\eta" />. This is simply the expected supra-threshold volume, divided by the expected number of clusters. The expected number of clusters <img class='tex' src="/wiki/images/math/b0ca0d9df95d65286632112b2c3f9cc1.png" alt="\psi_0" /> is estimated with the Euler characteristic (EC) (effectively the number of blobs minus the number of holes). This depends on the EC density for the statistic in question (with degrees of freedom <img class='tex' src="/wiki/images/math/959822faa03ebcea9210021baf302c54.png" alt="\nu" />) and the RESEL counts. The EC density is the expected EC per unit of <img class='tex' src="/wiki/images/math/5616208fafc6b96190c6b46e0b649a10.png" alt="D" />-dimensional volume of the SPM where the volume of the search is given by the RESEL counts. RESEL counts are a volume measure that has been normalized by the smoothness of the SPMs component error fields (<img class='tex' src="/wiki/images/math/444bc4156297541dbcd45dc59237d731.png" alt="\epsilon" />), expressed in terms of the full width at half maximum (FWHM). In this example equations for a sphere of radius <img class='tex' src="/wiki/images/math/444bc4156297541dbcd45dc59237d731.png" alt="\epsilon" /> are given. <img class='tex' src="/wiki/images/math/398b8c3e58e961cdea0fe626771f3243.png" alt="\Psi" /> denotes the cumulative density function for the statistic in question.</a>
Classical inference using SPMs can be of two sorts, depending on whether one knows where to look in advance. With an anatomically constrained hypothesis, about effects in a particular brain region, the uncorrected p-value associated with the height or extent of that region in the SPM can be used to test the hypothesis. With an anatomically open hypothesis (i.e. a null hypothesis that there is no effect anywhere in a specified volume) a correction for multiple dependent comparisons is necessary. The theory of random fields provides a way of adjusting the p-value that takes into account the fact that neighbouring voxels are not independent, by virtue of continuity in the original data. Provided the data are smooth the RFT adjustment is less severe (i.e. is more sensitive) than a Bonferroni correction for the number of voxels. As noted above RFT deals with the multiple comparisons problem in the context of continuous, statistical fields, in a way that is analogous to the Bonferroni procedure for families of discrete statistical tests. There are many ways to appreciate the difference between RFT and Bonferroni corrections. Perhaps the most intuitive is to consider the fundamental difference between an SPM and a collection of discrete t-values. When declaring a peak or cluster of the SPM to be significant, we refer collectively to all the voxels associated with that feature. The false positive rate is expressed in terms of peaks or clusters, under the null hypothesis of no activation. This is not the expected false positive rate of voxels. If the SPM is smooth, one false positive peak may be associated with hundreds of voxels. Bonferroni correction controls the expected number of false positive voxels, whereas RFT controls the expected number of false positive peaks. Because the number of peaks is always less than the number of voxels, RFT can use a lower threshold, rendering it much more sensitive. In fact, the number of false positive voxels is somewhat irrelevant because it is a function of smoothness. The RFT correction discounts voxel size by expressing the search volume in terms of smoothness or resolution elements (RESELS), see Fig.<a href="#F2">2</a>. This intuitive perspective is expressed formally in terms of differential topology using the Euler characteristic (Worsley et al. 1992). At high thresholds the Euler characteristic corresponds to the number peaks above threshold.
There are only two assumptions underlying the use of the RFT:
- The error fields (but not necessarily the data) are a reasonable lattice approximation to an underlying random field with a multivariate Gaussian distribution,
- These fields are continuous, with an analytic autocorrelation function.
In practice, for neuroimaging data, the inference is appropriate if 1) the threshold chosen to define the blobs is high enough such that the expected Euler characteristics is close to the number of blobs, which for cluster size tests would be around a Z score of three 2) the lattice approximation is reasonable, which implies a smoothness about three times the voxel size on each space axis, 3) the errors of the specified statistical model are normally distributed, which implies that the model is not mispecified.
A common misconception is that the autocorrelation function has to be Gaussian. It does not. The only way RFT might not be valid is if at least one of the above assumptions does not hold.
<a name="Anatomically_closed_hypotheses"></a>
Anatomically closed hypotheses
When making inferences about regional effects (e.g. activations) in SPMs, one often has some idea about where the activation should be. In this instance a correction for the entire search volume is inappropriate. However, a problem remains in the sense that one would like to consider activations that are 'near' the predicted location, even if they are not exactly coincident. There are two approaches one can adopt: pre-specify a small search volume and make the appropriate RFT correction (Worsley et al. 1996) or use the uncorrected p-value based on spatial extent of the nearest cluster (Friston 1997). This probability is based on getting the observed number of voxels, or more, in a given cluster (conditional on that cluster existing). Both these procedures are based on distributional approximations from RFT.
<a name="Anatomically_open_hypotheses_and_levels_of_inference"></a>
Anatomically open hypotheses and levels of inference
To make inferences about regionally specific effects the SPM is thresholded, using some height and spatial extent thresholds that are specified by the user. Corrected p-values can then be derived that pertain to various topological features of the excursion set (i.e. subset of the SPM above threshold):
- Set-level inference: the number of activated regions (i.e., number of connected subsets above some height and volume threshold),
- Cluster-level inference: the number of activated voxels (i.e., volume) comprising a particular connected subset (i.e., cluster),
- Peak-level inference: the height of maxima within that cluster.
These p-values are corrected for the multiple dependent comparisons and are based on the probability of obtaining <img class='tex' src="/wiki/images/math/2d043a3ca0862ca27fb2ff3a1eb2f1cf.png" alt="c" />, or more, clusters with <img class='tex' src="/wiki/images/math/524eecc1d5922a435e8e848094405caa.png" alt="k" />, or more, voxels, above a threshold <img class='tex' src="/wiki/images/math/1e0fbb458ac1325b073be7a4fad98fc8.png" alt="u" /> in an SPM of known or estimated smoothness. This probability has a reasonably simple form (see Fig.<a href="#F2">2</a> for details).
Set-level refers to the inference that the number of clusters comprising an observed activation profile is highly unlikely to have occurred by chance and is a statement about the activation profile, as characterized by its constituent regions. Cluster-level inferences are a special case of set-level inferences, that obtain when the number of clusters <img class='tex' src="/wiki/images/math/eafba6fb3a788835c0cc04808b379c77.png" alt="c = 1" />. Similarly peak-level inferences are special cases of cluster-level inferences that result when the cluster can be small (i.e. <img class='tex' src="/wiki/images/math/4ae87a89848522b017755603dc20693b.png" alt="k = 0" />). One usually observes that set-level inferences are more powerful than cluster-level inferences and that cluster-level inferences are generally more powerful than peak-level inferences. The price paid for this increased sensitivity is reduced localizing power. Peak-level tests permit individual maxima to be identified as significant features, whereas cluster and set-level inferences only allow clusters or sets of clusters to be identified. Typically, people use peak-level inferences and a spatial extent threshold of zero. This reflects the fact that characterizations of functional anatomy are generally more useful when specified with a high degree of anatomical precision.
Information extracted from here